by Paul Arora
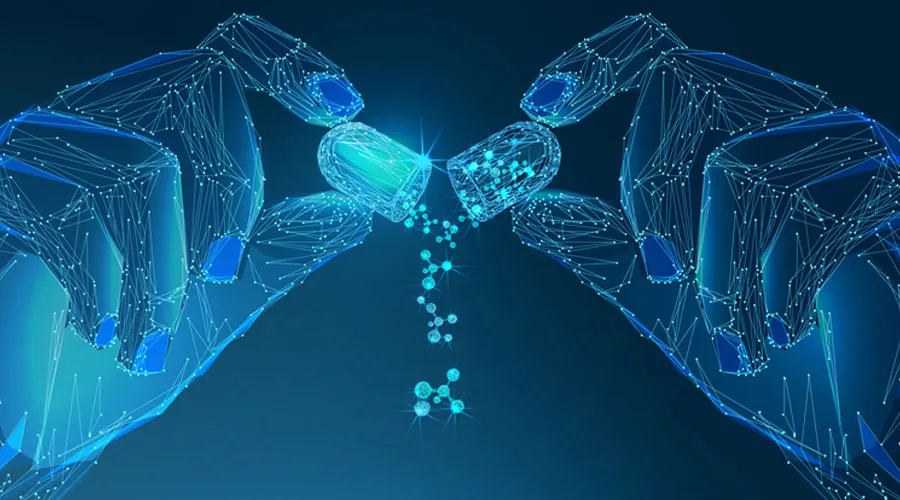
Greg Myers’ recent opinion piece highlights the promise of artificial intelligence in reshaping drug development and clinical trials but underscores the challenges posed by the immense complexity of biology and regulatory processes. While AI has demonstrated its utility in accelerating timelines and streamlining processes, it often falters when confronted with the intricate and interdependent systems that define human biology. This complexity demands more than just pattern recognition or data processing; it calls for a deeper understanding of cause-and-effect relationships.
This is where causal inference and causal AI stand out. Unlike conventional AI approaches, which excel at identifying correlations, causal AI focuses on uncovering and quantifying the relationships that drive outcomes. By leveraging real-world data (RWD) and embedding domain knowledge into visual graphs, causal AI allows researchers to address the underlying mechanisms of diseases, predict intervention effects, and minimize bias. Whether it’s optimizing patient selection for clinical trials or identifying new therapeutic uses for existing drugs, causal AI provides a structured, transparent approach to tackle the multifaceted nature of biomedical data.
The potential applications of causal AI are vast and already within reach. For instance, it can guide drug repurposing by linking mechanisms of action to disease pathways, significantly shortening development cycles by building on existing safety data. It can also personalize treatment plans by modeling how individual patient characteristics interact with treatment effects, providing tailored strategies in areas like oncology where variability is high. Furthermore, by identifying the true drivers of clinical outcomes, causal AI can optimize trial designs, making recruitment and execution more efficient while preserving the rigor required for regulatory approval.
Causal AI is not just another tool in the AI arsenal—it represents a necessary evolution for solving the complexity of modern drug development. At Inka Health, we are advancing software platforms that enable researchers and clinicians to apply causal AI in their workflows, facilitating smarter, faster, and more reliable decision-making in clinical and drug development. With the rapid growth of real-world data and the pressing need for actionable evidence, causal AI offers a scientific pathway forward—one grounded in precision and built for impact.
Comments